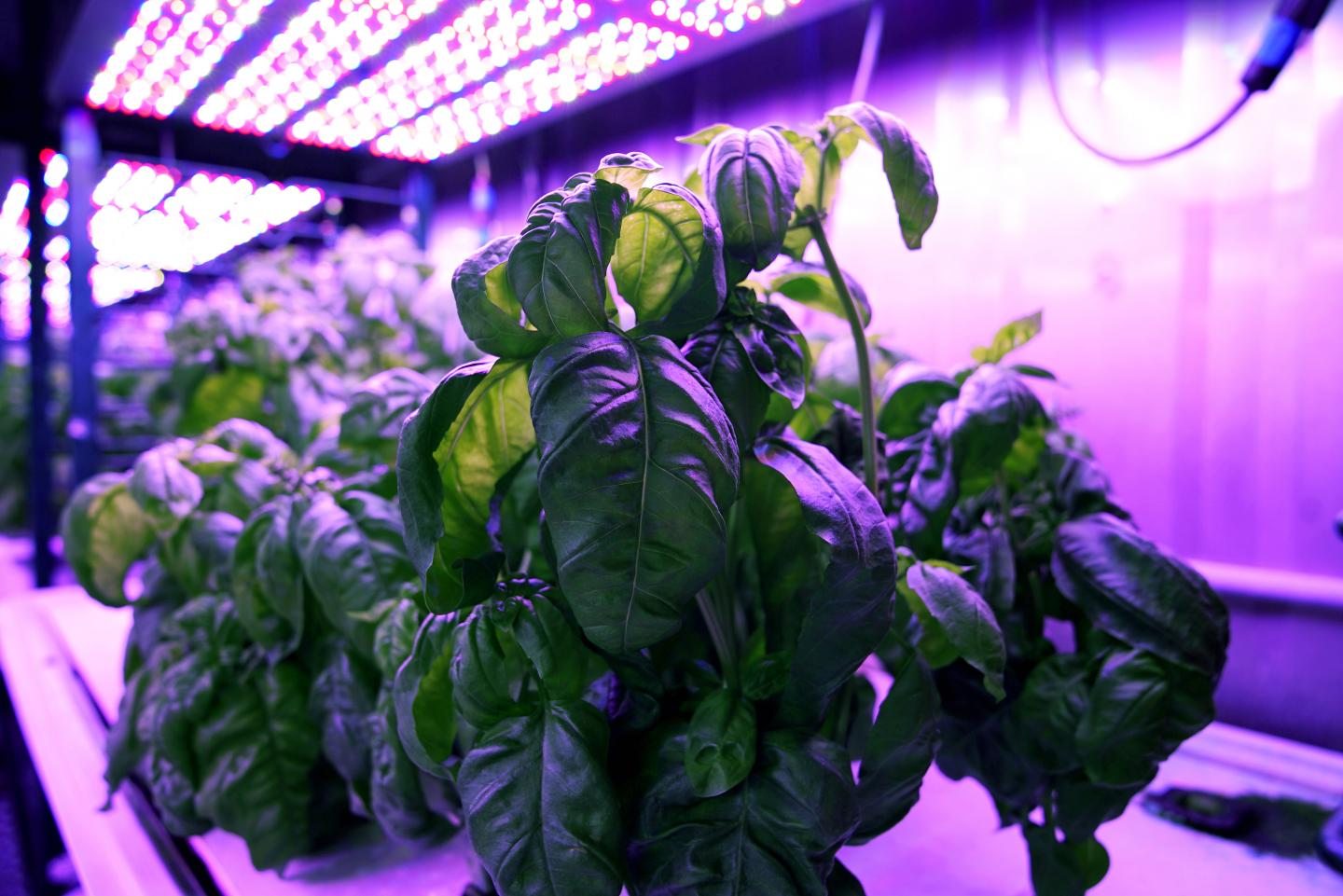
Researchers at MIT used artificial intelligence (AI) and machine learning to determine the best growing conditions to maximize taste the taste of basil.
The study, published in the journal PLOS One (citation below), involved a combination of cyber-agriculture, metabolomic phenotype (chemotype) measurements, and machine learning to maximize taste and other features of the herb.
“We’re really interested in building networked tools that can take a plant’s experience, its phenotype, the set of stresses it encounters, and its genetics, and digitize that to allow us to understand the plant-environment interaction,” explained Caleb Harper, principal research scientist at MIT’s Media Lab and director of the Open Agriculture (OpenAg) Initiative.
Two thousand basil plants were grown in hydroponic units, dubbed “food computers”, within modified shipping containers under a set of different conditions.
Environmental factors inside the containers, such as light and humidity, could be controlled automatically. This type of agriculture, known as controlled environmental agriculture, is still a niche market, but is growing fast, according to Harper.
The researchers collected data from the plants as they grew under different conditions. The researchers evaluated the the taste of basil using analytical chemistry techniques to measure the concentration of volatile compounds found in the plant’s leaves.
This data was then fed into machine-learning algorithms that the MIT and Cognizant (formerly Sentient Technologies) teams developed. The computer model then recommended the what growing conditions would produce the tastiest basil.
The model recommended exposing plants to light 24 hours a day generated for the best flavor. This type of insight would have been almost impossible to discover using traditional agricultural techniques.
“You couldn’t have discovered this any other way. Unless you’re in Antarctica, there isn’t a 24-hour photoperiod to test in the real world,” said John de la Parra, the research lead for the OpenAg group and an author of the study. “You had to have artificial circumstances in order to discover that.”
One of the goals of the MIT Initiative is to make all of OpenAg hardware, software, and data freely available for anyone to access. There’s been very limited sharing of information between firms that develop these types of controlled environmental agriculture facilities.
“There is a big problem right now in the agricultural space in terms of lack of publicly available data, lack of standards in data collection, and lack of data sharing,” Harper said.
“So while machine learning and artificial intelligence and advanced algorithm design have moved so fast, the collection of well-tagged, meaningful agricultural data is way behind. Our tools being open-source, hopefully they will get spread faster and create the ability to do networked science together.”
The team is now working on growing basil plants that have higher levels of compounds that could help to combat certain diseases such as diabetes.
“You can see this paper as the opening shot for many different things that can be applied, and it’s an exhibition of the power of the tools that we’ve built so far,” de la Parra said. “This was the archetype for what we can now do on a bigger scale.”
Citation
Johnson AJ, Meyerson E, de la Parra J, Savas TL, Miikkulainen R, Harper CB (2019) Flavor-cyber-agriculture: Optimization of plant metabolites in an open-source control environment through surrogate modeling. PLoS ONE 14(4): e0213918. https://doi.org/10.1371/journal.pone.0213918