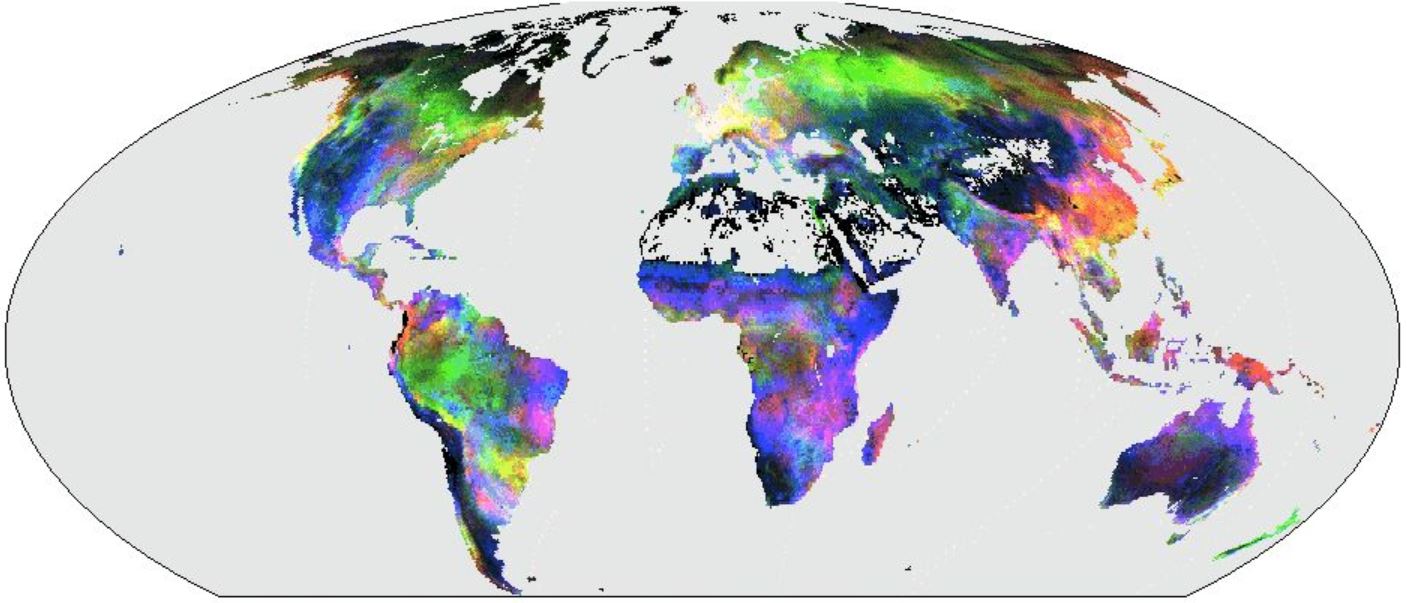
German scientists showed that deep learning techniques could significantly improve our understanding of Earth systems and climate. Deep learning’s potential has so far been just partially exhausted.
Scientists from Hamburg and Jena said that AI helps us better describe hurricanes, vegetation dynamics, and fire propagation.
The researchers wrote about their study and findings in the prestigious journal Nature (citation below). The authors were Markus Reichstein, Gustau Camps-Valls, Bjorn Stevens, Martin Jung, Joachim Denzler, Nuno Carvalhais, and Prabhat.
The authors wrote that, thanks to AI, Earth system models will be improved. The new models will be a combination of physical modeling and artificial intelligence.
Over the last couple of decades, researchers have used machine learning techniques mainly for static attributes. It has been possible to tackle more dynamic processes for some time now, thanks to sophisticated deep learning techniques.
Being able to tackle dynamic processes allows us, for example, to quantify global photosynthesis on land while considering short-term and seasonal variations at the same time.
Using deep learning techniques to deduce underlying laws
Markus Reichstein, Managing Director, of the Max Planck Institute for Biogeochemistry in Jena, Germany, said:
“From a plethora of sensors, a deluge of Earth system data has become available, but so far we’ve been lagging behind in analysis and interpretation.”
Joachim Denzler, Head of the Computer Vision Group of the Friedrich Schiller University, said:
“This is where deep learning techniques become a promising tool, beyond the classical machine learning applications such as image recognition, natural language processing or AlphaGo.”
Prof. Dr. Denzler is also a member of the Michael-Stifel-Center Jena for Data-driven and Simulation Science (MSCJ).
Applications include, for example, extreme events such as hurricanes and spreading fires. These complex processes are not only influenced by local conditions but also their spatial and temporal context. Applications also include atmospheric and ocean transport, vegetation dynamics, and soil movement.
Deep learning techniques to improve climate and Earth system models
According to a Max Planck Institute for Biogeochemistry press release:
“However, deep learning approaches are difficult. All data-driven and statistical approaches do not guarantee physical consistency per se, are highly dependent on data quality, and may experience difficulties with extrapolations. Besides, the requirement for data processing and storage capacity is very high.”
The authors discussed all these obstacles and requirements. They also developed a strategy to combine physical modeling with machine learning efficiently.
If we combine the two techniques, we create ‘hybrid models.’ We can use them, for example, for modeling ocean water motion to predict sea surface temperatures. The temperatures are modeled physically, but the movement of the ocean water is represented by machine learning techniques.
Prof. Dr. Reichstein added:
“The idea is to combine the best of two worlds, the consistency of physical models with the versatility of machine learning, to obtain greatly improved models,” Markus Reichstein further explains.”
The authors believe that their and hybrid modeling and deep-learning techniques will significantly improve the detection of extreme events. They will also improve the early warning of such events.
Artificial intelligence
Artificial intelligence (AI) refers to software that makes machines think and behave like humans. Deep learning is part of a broader family of machine learning methods.
With deep learning, neural networks learn from large amounts of data. One of AI’s features is its capacity for self-learning. In other words, to learn from large amounts of data without human help. Humans also have this ability.
Citation
“Deep learning and process understanding for data-driven Earth system science,” Markus Reichstein, Gustau Camps-Valls, Bjorn Stevens, Martin Jung, Joachim Denzler, Nuno Carvalhais, and Prabhat. Nature, volume 566, pages 195–204 (2019). DOI: https://doi.org/10.1038/s41586-019-0912-1.